Hello Nature readers, would you like to get this Briefing in your inbox free every week? Sign up here.
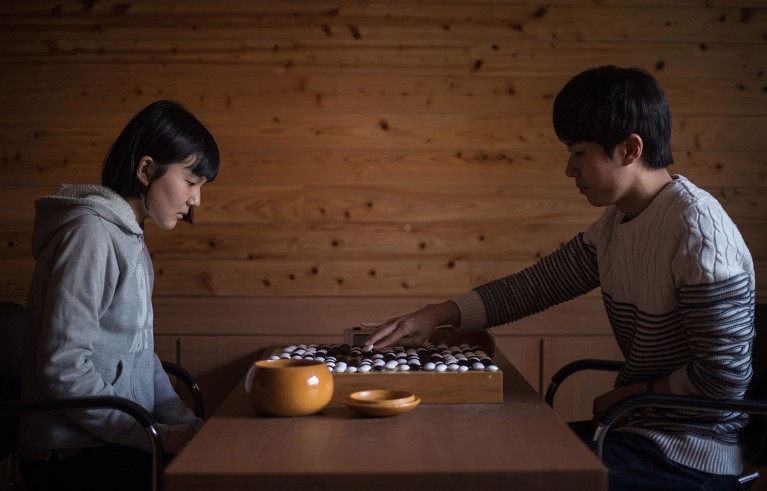
The board game Go is a high-profile test of machine-learning capabilities.Credit: Ed Jones/AFP via Getty
KataGo, a Go-playing AI system that can beat the world’s best human players, can be defeated by adversarial bots. These bots aren’t themselves good at the game — even amateurs can beat them — but they excel at feeding KataGo with inputs that cause it to make mistakes. Strategies for fortifying KataGo against such adversarial attacks mostly failed. “If we can’t solve the issue in a simple domain like Go, then in the near-term there seems little prospect of patching similar issues like jailbreaks in ChatGPT,” says AI researcher and study co-author Adam Gleave.
Reference: arXiv preprint (not peer reviewed)
A chatbot with the persona of a drag queen can answer delicate questions about sexual health in an empathetic, non-judgmental way. Out of the thousands of people who used the tool to supplement their in-person care, almost 80% chose the drag version over a standard chatbot. “Drag queens are about acceptance and taking you as you are,” says Whitney Engeran-Cordova from the AIDS Healthcare Foundation.
EvolutionaryScale’s system ESM-3 has generated proteins inspired by green fluorescent protein (GFP), a biotechnology workhorse used to make other proteins visible. One of the ESM-3 proteins glows as bright as a natural GFP. What makes it unusual is that it shares less than 60% of its building-block sequence with the most closely related fluorescent protein in the model’s training data. ESM-3 is one of the first biological models that allows researchers to design new proteins by simply writing down the properties and functions they want. “It’s going to be one of the AI models in biology that everybody’s paying attention to,” says computational biologist Anthony Gitter.
Reference: bioRxiv preprint (not peer reviewed)
An AI system can read a macaque’s brain activity and reconstruct with remarkable accuracy what the animal was looking at. Key to the model’s success are predictive attention mechanisms, essentially the ability to learn which parts of the brain it should pay most attention to. The monkey’s brain activity had been recorded with implanted electrodes, but the algorithm could also reconstruct images from MRI scans, albeit with much less accuracy. The researchers hope to eventually reverse the process: stimulate the brain to restore vision.
New Scientist | 3 min read (paywall)
Reference: bioRxiv preprint (not peer reviewed)
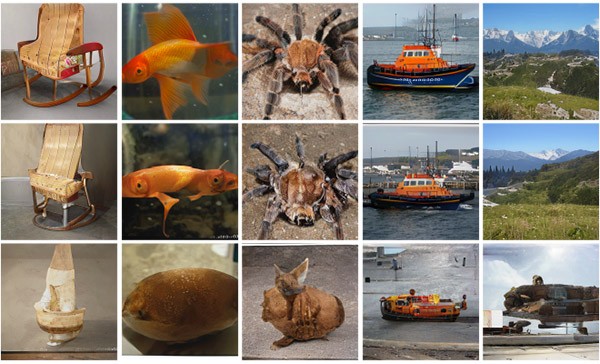
The original, AI-generated images (top row) can be reconstructed from brain recordings fairly accurately by an AI system with a predictive attention mechanism (middle row) — but less so by a system without this mechanism (bottom row). (Thirza Dado et al./bioRxiv (CC BY 4.0))
Features & opinion
Loss functions provide a mathematical measure of wrongness: they tell researchers how well their AI algorithms are working. There are dozens of off-the-shelf functions. But choosing the wrong one, or handling it badly, can create algorithms that blatantly contradict human observations or obscure experiments’ central results. Programming libraries such as PyTorch and scikit-learn allow scientists to easily swap and trial functions. A growing number of scientists are creating their own loss functions. “If you’re in a situation where you believe that there are probably errors or problems with your data … then it’s probably a good idea to consider using a loss function that’s not so standard,” says machine-learning researcher Jonathan Wilton.
Universal AI agents should be able to do complex tasks without needing to be micromanaged — from booking your holidays to sorting out work priorities. Right now, AI agents are at the state self-driving cars were more than a decade ago, says AI startup founder Kanjun Qiu. We have some highly specialized agents such as AlphaGo, but general ones remain clumsy and unreliable. One problem is the limited amount of data current AI models can take into account at one time. Another is their inability to reason, which is key to operating in our complex and ambiguous world.
MIT Technology Review | 7 min read
While voice assistants such as Apple’s Siri or Amazon’s Alexa retain a robotic drawl, ChatGPT’s ‘Sky’ voice — now discontinued because it sounded eerily similar to actor Scarlett Johansson — sounded natural, soothing and had a sexy edge, writes critic Amanda Hess. “She sounded like she was game for anything.” Filmmakers have long used this persona of an empathetic and compliant woman to make people feel at ease with robots — tech firms want to do the same, Hess argues. “What does artificial intelligence sound like? It sounds like crisis management.”
The New York Times | 7 min read
Image of the week
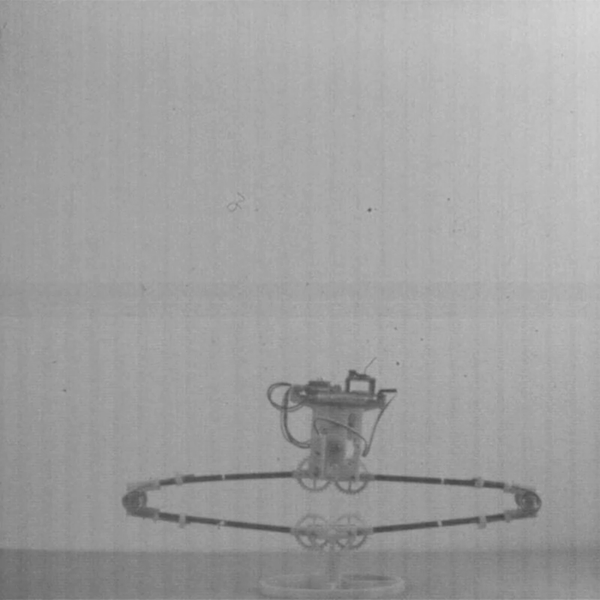
Dr John Lo/University of Manchester
Although this small robot barely jumps 2 metres high, it demonstrates how to convert around 50% of stored energy into vertical movement. Researchers discovered that jumping robots often launch themselves too early, before fully releasing the stored spring energy. Removing inefficiencies could increase the 30-metre robot jump height record four-fold, the team theorizes. (Interesting Engineering | 3 min read)
Reference: Mechanism and Machine Theory paper