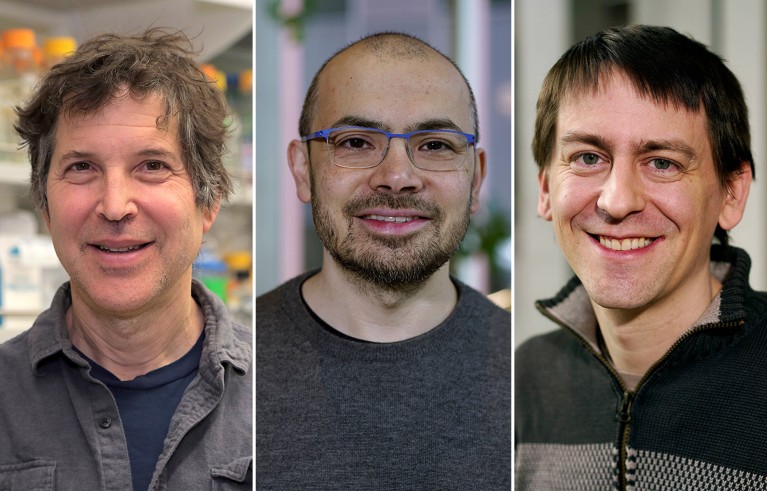
David Baker, Demis Hassabis and John Jumper (left to right) won the chemistry Nobel for developing computational tools that can predict and design protein structures.Credit: BBVA Foundation
For the first time — and probably not the last — a scientific breakthrough enabled by artificial intelligence has been recognized with a Nobel Prize. The 2024 chemistry Nobel was awarded to John Jumper and Demis Hassabis at Google DeepMind in London, for developing a game-changing AI tool for predicting protein structures called AlphaFold, and David Baker, at the University of Washington in Seattle, for his work on computational protein design, which has been revolutionized by Al in recent years.
What’s next for AlphaFold and the AI protein-folding revolution
The impact of AlphaFold, which was unveiled just a few years ago, has been nothing short of transformative. The tool has made protein structures — often, but not always, highly accurate ones — available to researchers at the touch of a button, and enabled experiments that were unimaginable a decade ago. Biologists now talk about an era ‘before AlphaFold’ and one after.
“It has long been a dream to learn to predict the three-dimensional structure of proteins from knowing their amino acid sequences … for several decades, this was considered impossible,” said Nobel committee chair Heiner Linke, who researches nanoscience at Lund University in Sweden, during the prize announcement. This year’s laureates “have cracked the code”, he added. The three winners share a prize pot of 11 million Swedish kronor (US$1 million).
Award-winning AI
DeepMind debuted AlphaFold in 2018, when it won a biennial protein structure prediction contest called the Critical Assessment of Protein Structure Prediction (CASP). But it was the second iteration of the deep learning neural network, revealed in late 2020, that set off an earthquake in the life sciences.
Many of AlphaFold2’s predictions at CASP were so accurate as to be indistinguishable from experimentally-solved protein structures. This prompted CASP co-founder and computational biologist John Moult, at the University of Maryland in College Park, to declare in 2020 that “in some sense, the problem is solved”.
Hassabis, DeepMind’s co-founder and CEO, and Jumper, head of the AlphaFold team, led the development of AlphaFold2. To predict protein structures, the neural network incorporates similar structures from databases of hundreds of thousands of experimentally-solved structures and millions of sequences from related proteins — which hold information about their shapes.
How to win a Nobel prize: what kind of scientist scoops medals?
In 2021 DeepMind made AlphaFold2’s underlying code freely available, along with the data needed to train the model. An AlphaFold database, created with the European Molecular Biology Lab’s European Bioinformatics Institute in Hinxton, UK, now holds the structures of almost all the proteins from every organism represented in genetic databases, some 214 million predictions in total. This year, the company unveiled a third version of AlphaFold, which can model other molecules that interact with proteins such as drugs.
The revolution that Jumper, Hassabis and their colleagues unleashed is still in its early days, and AlphaFold’s full impact on science may not be known for years. Already, the tool is helping scientists make new insights.
One pioneering team used the tool, along with experimental data, to map the nuclear pore complex, one of our cells’ largest machines that transports molecules into and out of the nucleus. Last year, two teams mined the entire AlphaFold Database to uncover the darkest corners of the protein universe, identifying new families of proteins and folds and surprising connections in the machinery of life.
Many researchers hope that AlphaFold and other AI tools it has inspired will transform medicine, but it is not yet clear how, or indeed whether, AlphaFold will streamline the costly and multi-step process of developing safe new drugs.
Creating new proteins
More than a decade before DeepMind started working on AlphaFold, computational biophysicist David Baker, at the University of Washington in Seattle, and his colleagues developed software tools for modelling protein structures using physical principles called Rosetta. The tool had early success designing novel proteins.
Over the years, Baker’s team applied Rosetta to predicting protein structure — it has been among the top entries at numerous CASPs, prior to AlphaFold’s recent hegemony — as well as designing novel proteins such as enzymes and self-assembling protein nanoparticles.
Medicine Nobel awarded for gene-regulating ‘microRNAs’
When AlphaFold2 was announced — but not yet released — Baker and his team, including computational chemist Minkyung Baek, now at Seoul National University in South Korea, set out to understand the software and apply some of its tricks to a previous AI-based version of Rosetta. The first version of the resulting RoseTTAFold network performed nearly as well as AlphaFold2. Since 2021, both networks have been continually improved by their developers and other scientists to tackle fresh challenges, such as predicting the structure of complexes of multiple different interacting proteins.
In recent years, Baker’s team have been especially prolific in applying machine learning to his lab’s raison d’etre: creating new proteins never seen before in nature. A tool recently developed by Baker’s team that melds RoseTTAFold with image-generating diffusion neural networks has led to a step-change in researchers’ capacity to design proteins.
Although computational tools such as AlphaFold aren’t a replacement for experimental studies, they are an accelerator, scientists say. “This is going to empower a new generation of molecular biologists to ask more advanced questions,” CASP judge Andrei Lupas, an evolutionary biologist at the Max Planck Institute for Developmental Biology in Tübingen, Germany, told Nature in 2020.
This is a breaking news story that will be updated throughout the day.
Additional reporting by Helena Kudiabor.